
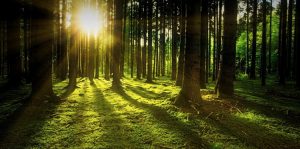
In this manuscript, we seek to optimize random forest prediction performance using combinations of variable screening, class balancing, weighting, and hyperparameter tuning. It has also been shown to handle challenges arising from small sample sizes. RF has many advantages: it is fast in both model training and evaluation, is robust to outliers, can capture complex nonlinear associations, cope with class imbalance data, and produces competitive performance for high dimensional data.
RANDOM FOREST HYPERPARAMETER TUNING TRIAL
For example, RF have been used to recognize cancer-associated biomarkers from clinical trial data, to predict protein-protein interactions, and to identify informative genes for a disease from microarray gene expression data. Random forests are a popular machine learning method that have been increasingly used in biomedical applications. Most conventional machine learning methods tend to be unsuccessful in situations with small sample sizes because the methods require a substantial amount of training data. Typically, subjects in the cohort are classified into several strata based on the cohort information, and then a subset of subjects is randomly sampled without replacement from each stratum (see Additional file 1: Section D for a more detailed explanation.) Studies using the two-phase sampling designs often have a small number of disease endpoints and a high cost associated with measuring biomarkers such that only a small representative subset of controls have biomarker measurements. Two-phase sampling is a method to design substudies on selected subjects from a cohort to avoid measuring expensive covariates for every participant in the cohort. However, machine learning methods have not been widely adopted in the context of prevention clinical trials using two-phase sampling designs. Many machine learning methods have been used with great success in solving problems as diverse as early prognosis and diagnosis of a cancer type, identifying rare disease, and prediction of infectious disease risk. Prediction of a binary disease outcome from a collection of clinical covariates and biomarker measurements is a common task in biomedical studies. In addition, stacking random forests and simple linear models can offer improvements over random forests. In small datasets from two-phase sampling design, variable screening and inverse sampling probability weighting are important for achieving good prediction performance of random forests. We further show that random forests under-perform generalized linear models for some subsets of markers, and prediction performance on this dataset can be improved by stacking random forests and generalized linear models trained on different subsets of predictors, and that the extent of improvement depends critically on the dissimilarities between candidate learner predictions. Hyperparameter tuning is ineffective in situations with small sample sizes. The impact of the weighting similarly depends on whether variable screening is applied. Our experiments show that while class balancing helps improve random forest prediction performance when variable screening is not applied, class balancing has a negative impact on performance in the presence of variable screening. Using an immunologic marker dataset from a phase III HIV vaccine efficacy trial, we seek to optimize random forest prediction performance using combinations of variable screening, class balancing, weighting, and hyperparameter tuning.
RANDOM FOREST HYPERPARAMETER TUNING PLUS
Specify the expected improvement plus function as the acquisition function and suppress printing the optimization information.While random forests are one of the most successful machine learning methods, it is necessary to optimize their performance for use with datasets resulting from a two-phase sampling design with a small number of cases-a common situation in biomedical studies, which often have rare outcomes and covariates whose measurement is resource-intensive. 'NumPredictorstoSample',params.numPTS) Įnd Minimize Objective Using Bayesian Optimizationįind the model achieving the minimal, penalized, out-of-bag quantile error with respect to tree complexity and number of predictors to sample at each node using Bayesian optimization. 'OOBPrediction', 'on', 'MinLeafSize',params.minLS. RandomForest = TreeBagger(300,X, 'MPG', 'Method', 'regression'. X is a table % and params is an array of OptimizableVariable objects corresponding to % the minimum leaf size and number of predictors to sample at each node. %oobErrRF Trains random forest and estimates out-of-bag quantile error % oobErr trains a random forest of 300 regression trees using the % predictor data in X and the parameter specification in params, and then % returns the out-of-bag quantile error based on the median.
